Assortment Optimization with Consumer Preference Learning
Optimization based on consumer preference for a major international beverage company
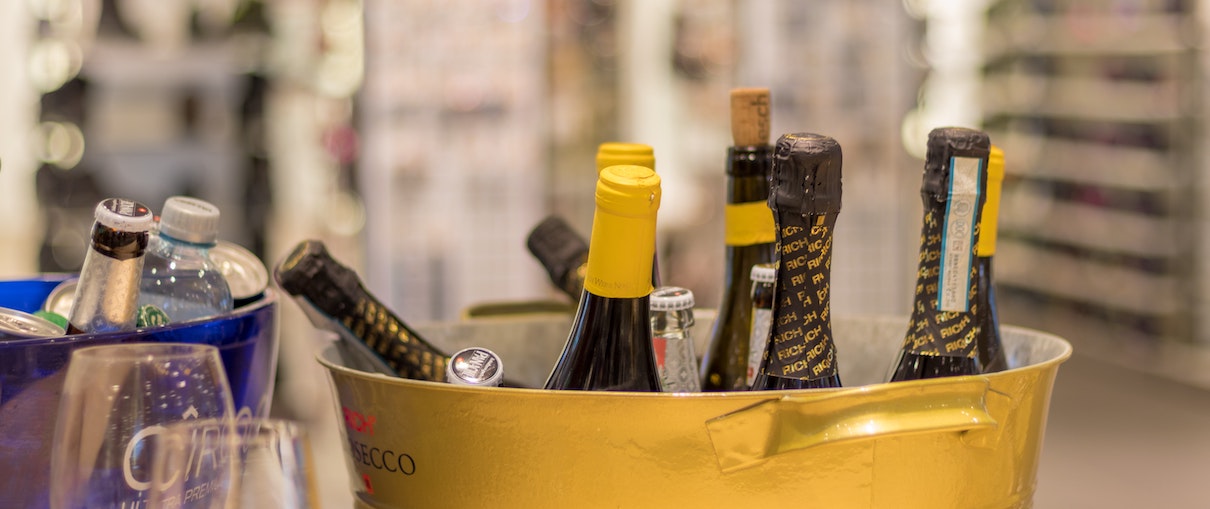
Product assortment optimization needs better understanding of consumer preferences
Given the vastly different mixes of consumer types in stores across the country, there is no simple one-size-fits-all product assortment. Instead, a different mix of products is selected per-store. Historically, this has been done based purely on simple geographical features such as the store's state and whether it is designated as urban or rural. Knowing that this leaves a lot on the table, we collaborated to develop a data-driven assortment for each store based on consumer preference learning and optimization.
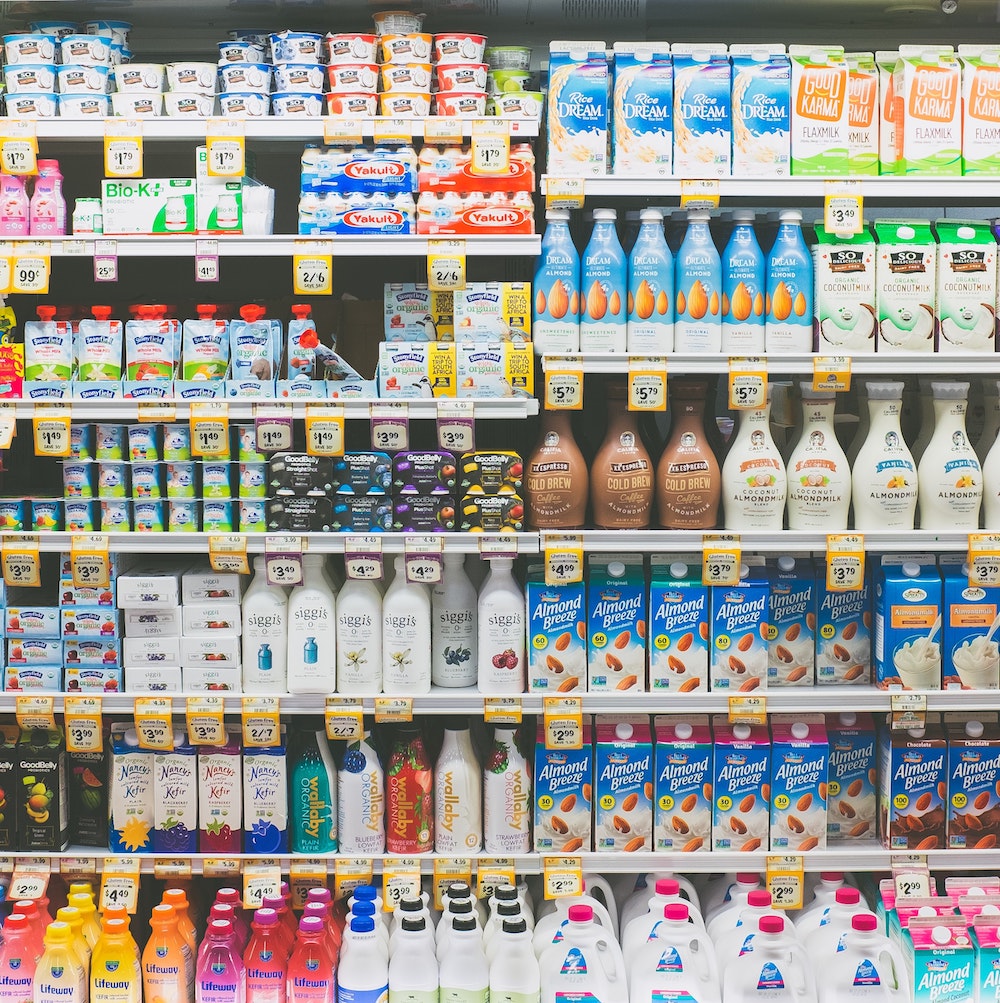
Consumer preference groups from historical data
-
Learning preferences from purchase history
We developed an optimization model that would allow us to learn customer preferences from the observed sales data. This model was embedded inside the Optimal Trees framework to train a decision tree that would simultaneously learn the consumer preferences in each store and segment the stores into groups with similar preferences.
-
Meaningful homogenous consumer groups
Each group is comprised of stores with similar consumer preferences, and is defined by simple characteristics such as the store's type, size, and local demographic information. The interpretability of the tree allowed the market experts to validate these segments against their intuition.
Example tree for consumer preference segmentation
Optimized assortment per group leads to increased sales
Our partner conducted a pilot study in Texas to implement these optimized per-store product assortments, and estimated an average 1% increase in revenue, with some stores seeing significantly higher increases in sales. They are currently planning on further testing both in other states and outside the United States.
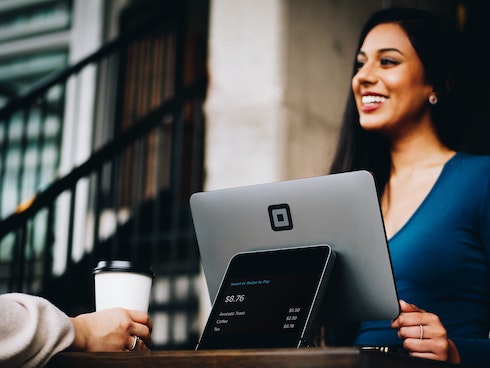
Unique Advantage
Why is the Interpretable AI solution unique?
-
Meaningful differentiation
Market experts can understand the features that are meaningful for grouping the stores based on similar consumer preference profiles.
-
Data-informed optimization
A better grouping of homogenous stores leads to improved assortment optimization in each store, compared to grouping based on geographical features alone.