Pricing for Real Estate Auctions
Optimizing pricing recommendations to increase sales rates and revenue
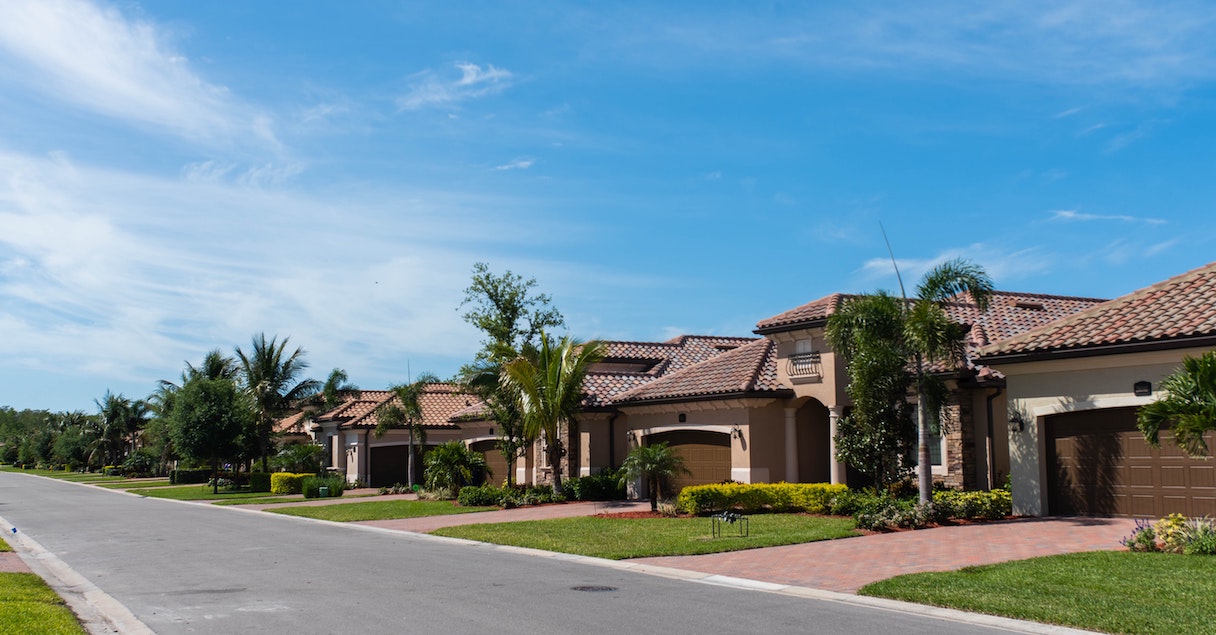
Pricing assets with valuation models alone is insufficient
To address this problem of pricing in dynamic online marketplace, we partnered with one of the largest online real-estate platforms to build a market-driven dynamic pricing model. The model would inform sellers on optimal pricing decisions for their properties, with the goal of maximizing overall revenue.
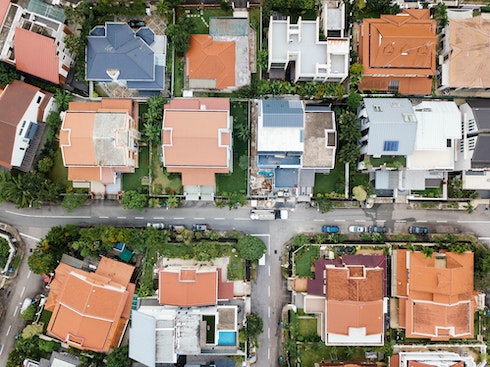
Accurate and intuitive sellability model as the basis for pricing
-
High accuracy
The model predicted the sale probability in unseen data with over 80% precision, better than human portfolio managers.
-
Meaningful reasons for sales outcomes
The prediction logic was presented to and validated by experts, with their feedback incorporated to improve the model interpretability and performance.
-
Up-to-date market conditions
The model is updated with data under current market conditions to ensure it is always applicable and relevant.
Illustrative Optimal Classification Tree to predict probability of sales
Dynamic multi-run pricing strategy
Using dynamic programming and outputs from the sellability model, we build a life-time value optimization model that recommends a sequence of prices, which is accompanied by the reasoning at each run.
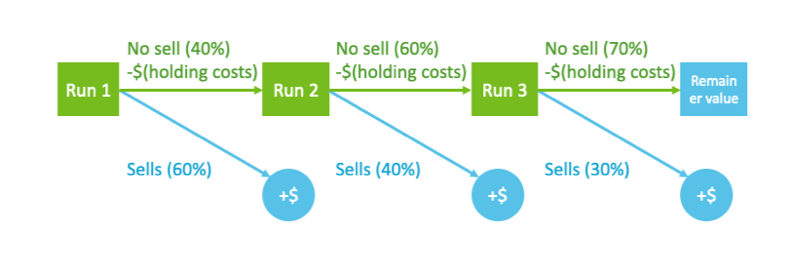
Schema for the life-time value optimization model
Increased sales rate and revenue for porfolios
In a pilot study, the client identified that the new pricing tool could result in 18% additional sales and 24% overall higher revenue. A joint white paper is coming out soon that details the methodology and impact at the client site.
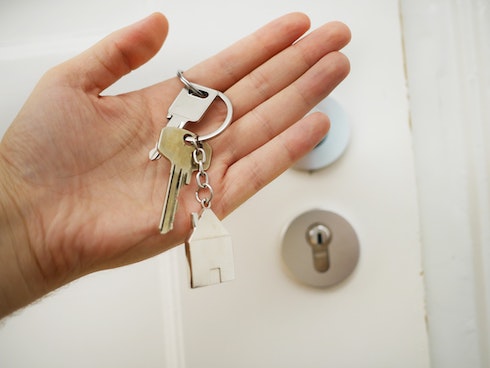
Unique Advantage
Why is the Interpretable AI solution unique?
-
Market-driven sales probability
As opposed to just another valuation model, this model learns market appeal and sellability from historical transactions
-
Communication with evidence
The tool provides portfolio managers with reasons that support price changes
-
Formal optimization
Rigorous optimization to derive the optimal pricing strategy in a complex multi-period process