Pricing of Exchange-Traded Assets
Predicting the fair market price of a financial instrument
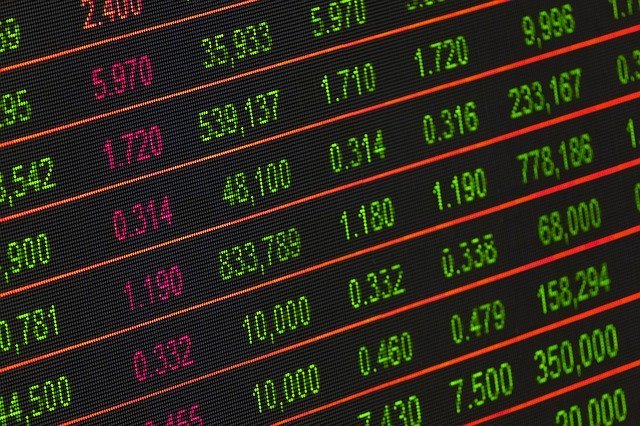
Current pricing methods are not robust to all market conditions
Our client, a large investment bank, needs to price the value of its assets in real-time,
either to hedge its own positions or to provide quotes to third parties.
The traditional approach to this problem is to approximate the fair value with a fixed, simple price formula. This approach performs well on average, but yields poor value estimates in adverse market conditions.
We used Optimal Policy Trees to design a new pricing methodology that better leverages the singularities of every market situation, while maintaining interpretability.
The traditional approach to this problem is to approximate the fair value with a fixed, simple price formula. This approach performs well on average, but yields poor value estimates in adverse market conditions.
We used Optimal Policy Trees to design a new pricing methodology that better leverages the singularities of every market situation, while maintaining interpretability.
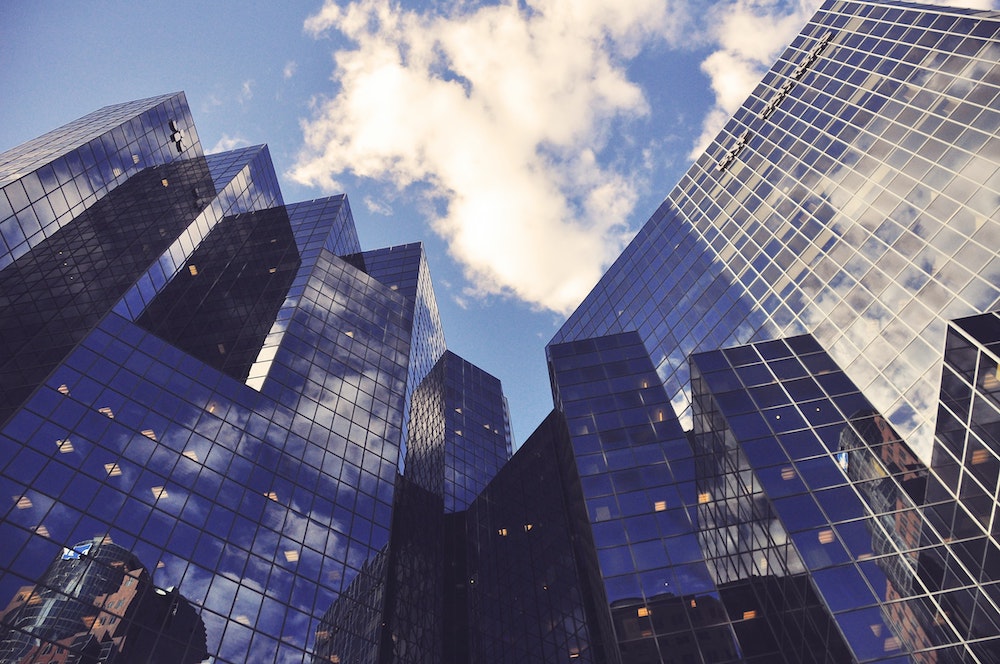
Using the right price at the right moment
Policy Trees are a class of prescriptive algorithms that can be applied
when we have full information about the outcome associated with each possible choice.
In collaboration with traders and market experts, we first generated a series of meaningful price formulae, each one being designed to perform well in a specific market scenario. At every timestep, we then monitored traditional market signals and observed which of the generated prices was closest to the true market price.
Leveraging all the available market indicators at each of those timesteps, we used an Optimal Policy Tree to learn which conditions are best suited for what price.
In collaboration with traders and market experts, we first generated a series of meaningful price formulae, each one being designed to perform well in a specific market scenario. At every timestep, we then monitored traditional market signals and observed which of the generated prices was closest to the true market price.
Leveraging all the available market indicators at each of those timesteps, we used an Optimal Policy Tree to learn which conditions are best suited for what price.
Illustrative Optimal Policy Tree prescribing what type of price to use
Easy to understand, easy to adopt
During the modeling phase, the simple flow of our trees allowed domain experts to quickly understand the market dynamics at stake for pricing.
As a result, we were able to improve the model with new features at a much higher pace than we would with a black-box method.
By the end of the project, our model was consistently outperforming the bank's previous approach to pricing by up to 2%, and its high level of transparency facilitated its adoption by the management team.
By the end of the project, our model was consistently outperforming the bank's previous approach to pricing by up to 2%, and its high level of transparency facilitated its adoption by the management team.
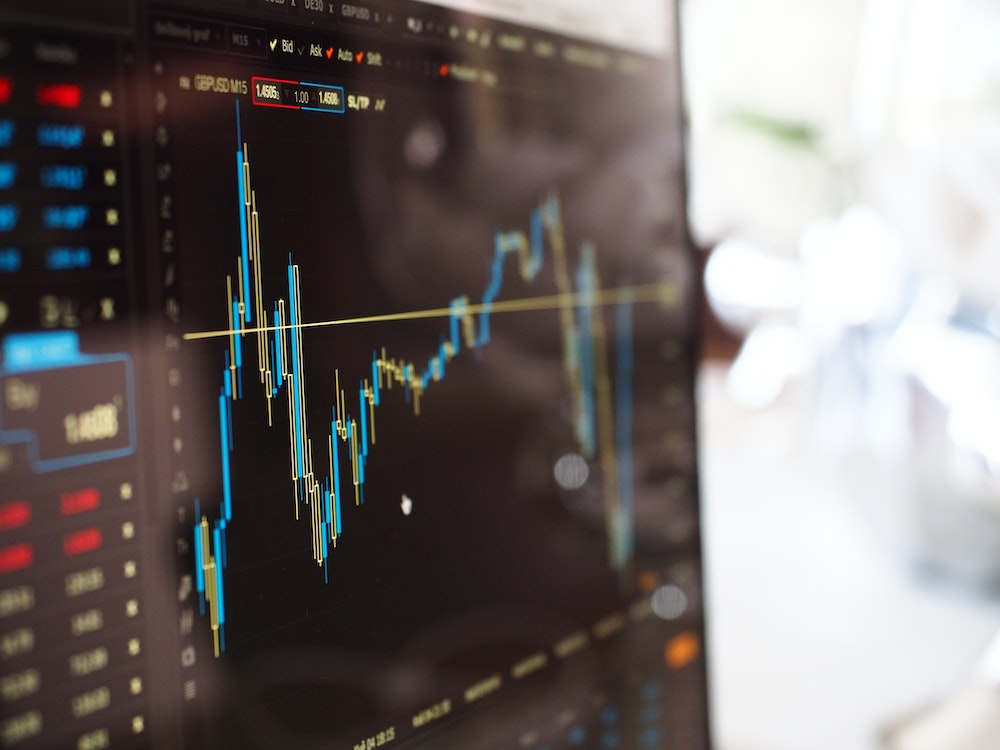
Unique Advantage
Why is the Interpretable AI solution unique?
-
A novel approach to policy learning
Optimal Policy Trees are the first scalable and optimal solution to this class of problem
-
Building on the knowledge of experts
At every stage of model development, the transparency of our model facilitates collaboration with domain experts to improve and audit the final results