Optimizing Data Acquisition
Identifying the right data to acquire using Optimal Feature Selection
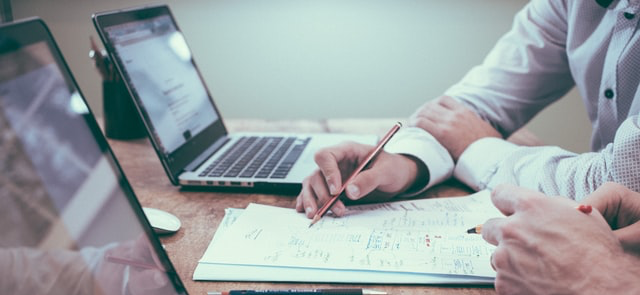
Acquiring data to train a machine learning model
- Will the new data actually improve performance?
- How much new data do we need?
- How to weigh the trade-off between the cost of acquisition and the performance boost?
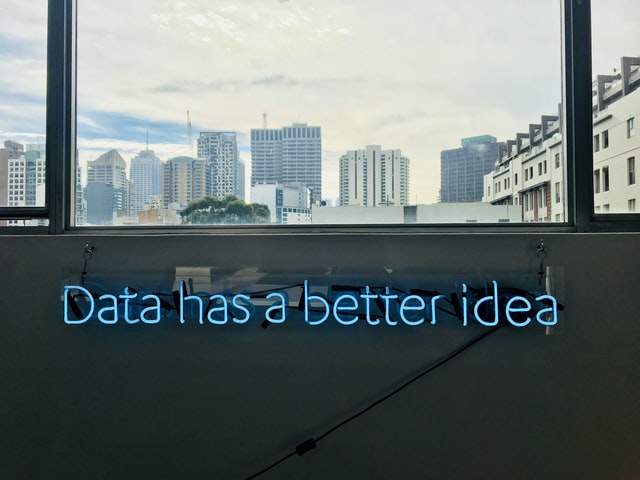
Credit card spending patterns for insurance risk prediction
-
Selecting the optimal set of features...
Acquiring hundreds of features (such as healthcare expenditures, credit line information, etc.) can quickly become expensive, and most of these features will not carry sufficient predictive power to be used by our final machine learning model. Optimal Feature Selection can select the best set of features that our partner will need to acquire going forward.
-
...by only acquiring data for a subset of households
In order to identify this optimal set of credit card features, we only needed to purchase all available features for a small subset of households. Optimal Feature Selection can learn from limited data, thus further restricting research and development costs.
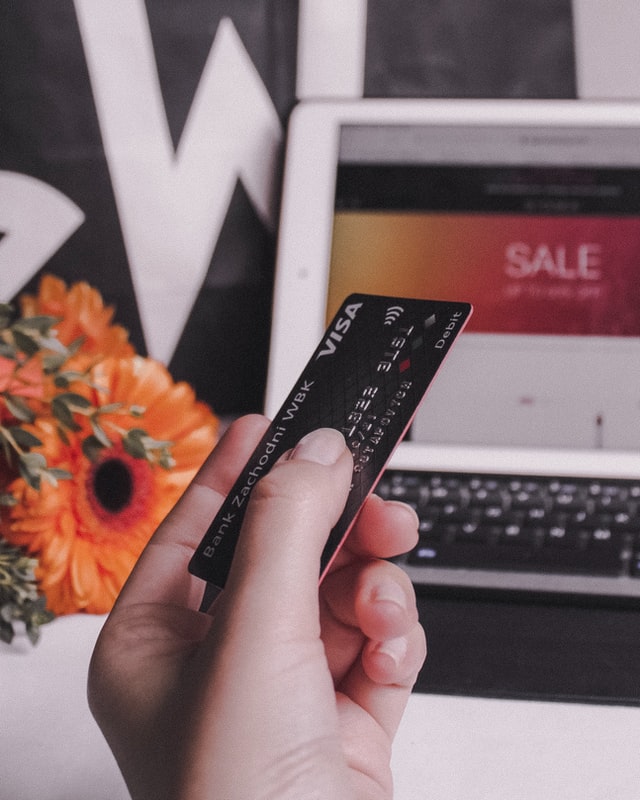
Optimizing data acquisition cost
Increased transparency in handling sensitive data
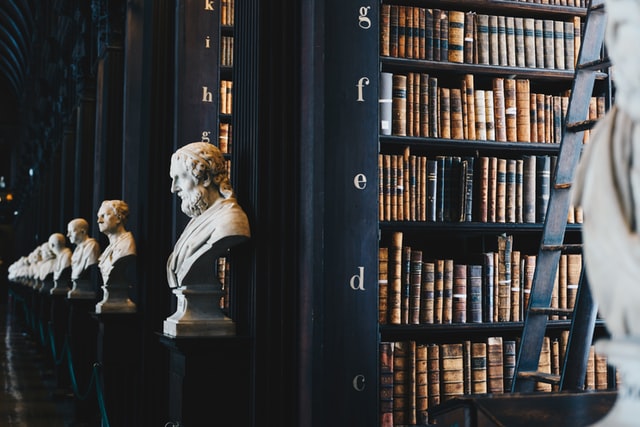
Unique Advantage
Why is the Interpretable AI solution unique?
-
Cost reduction
Identify the right features to acquire and thus eliminate unnecessary data acquisition costs
-
Reduced data engineering burden
Less data to acquire also eliminates the need for complex data engineering pipelines
-
Simple and transparent
Fewer features selected means a simpler, more transparent, and auditable model