Interpretable Clustering for Demand Forecasting
Identifying similar cohorts to predict product demand more accurately
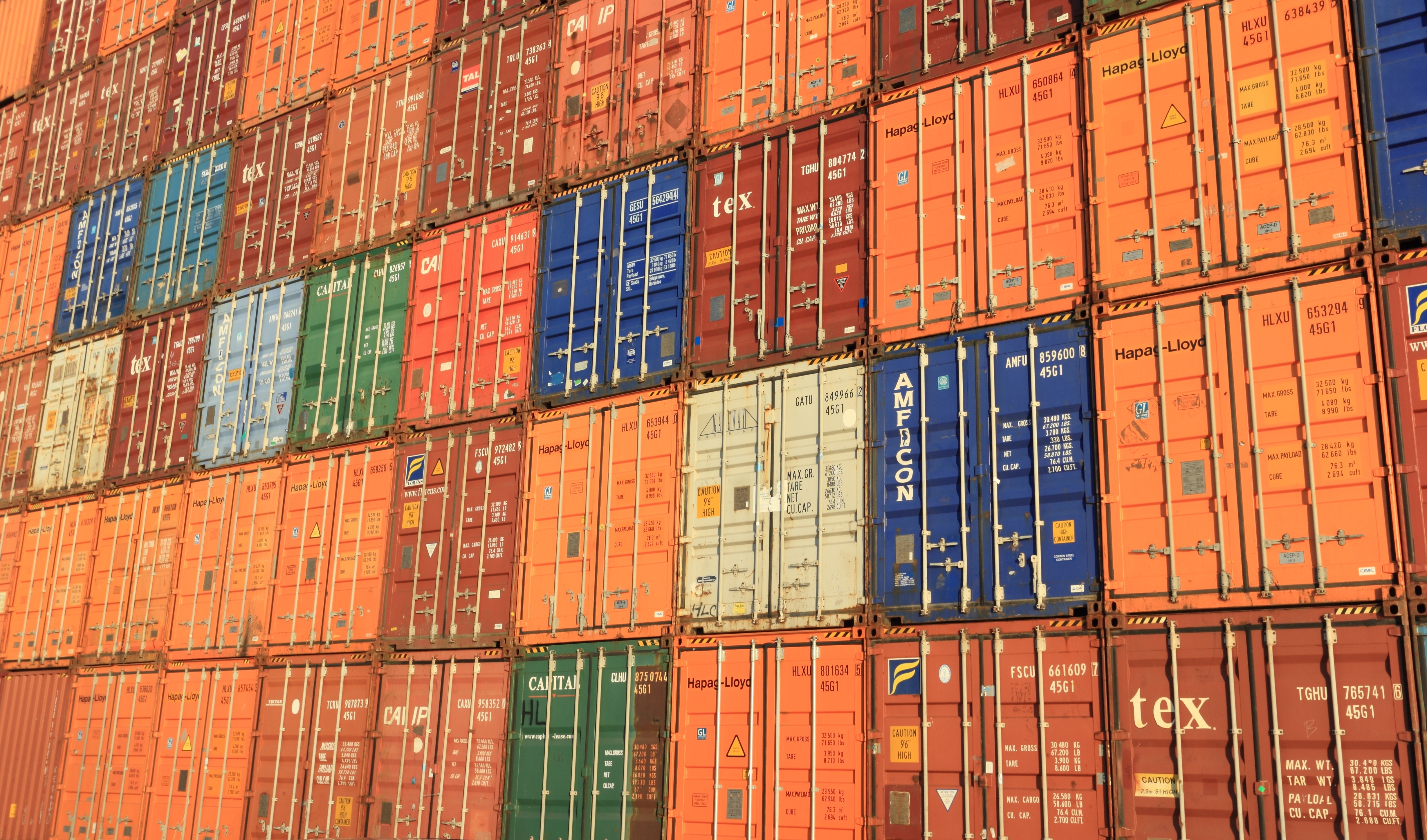
Demand forecasts are key in the stategic planning of any business
Additionally, their data scientists struggled to improve the model because they couldn't identify where the models systematically underperformed or outperformed. This put their team at risk of committing the same mistakes repeatedly.
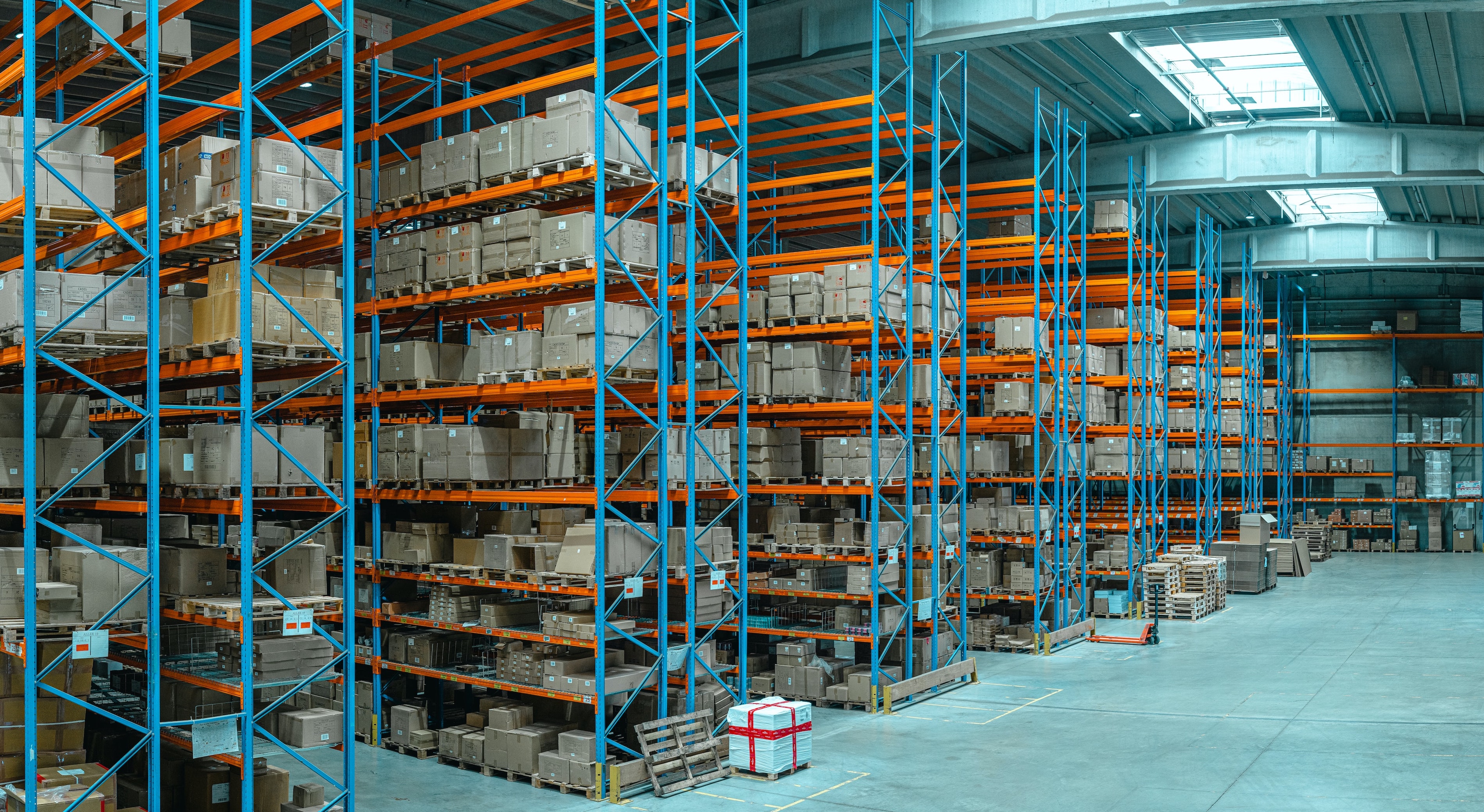
Interpretable Clustering identifies cohorts with similar characteristics
We define a cluster as the collection of observations falling into a leaf of the classification model, as by definition they share similar characteristics (the features used as splits by the tree). Then we train Optimal Regression Trees to generate interpretable demand forecasts for each cluster.
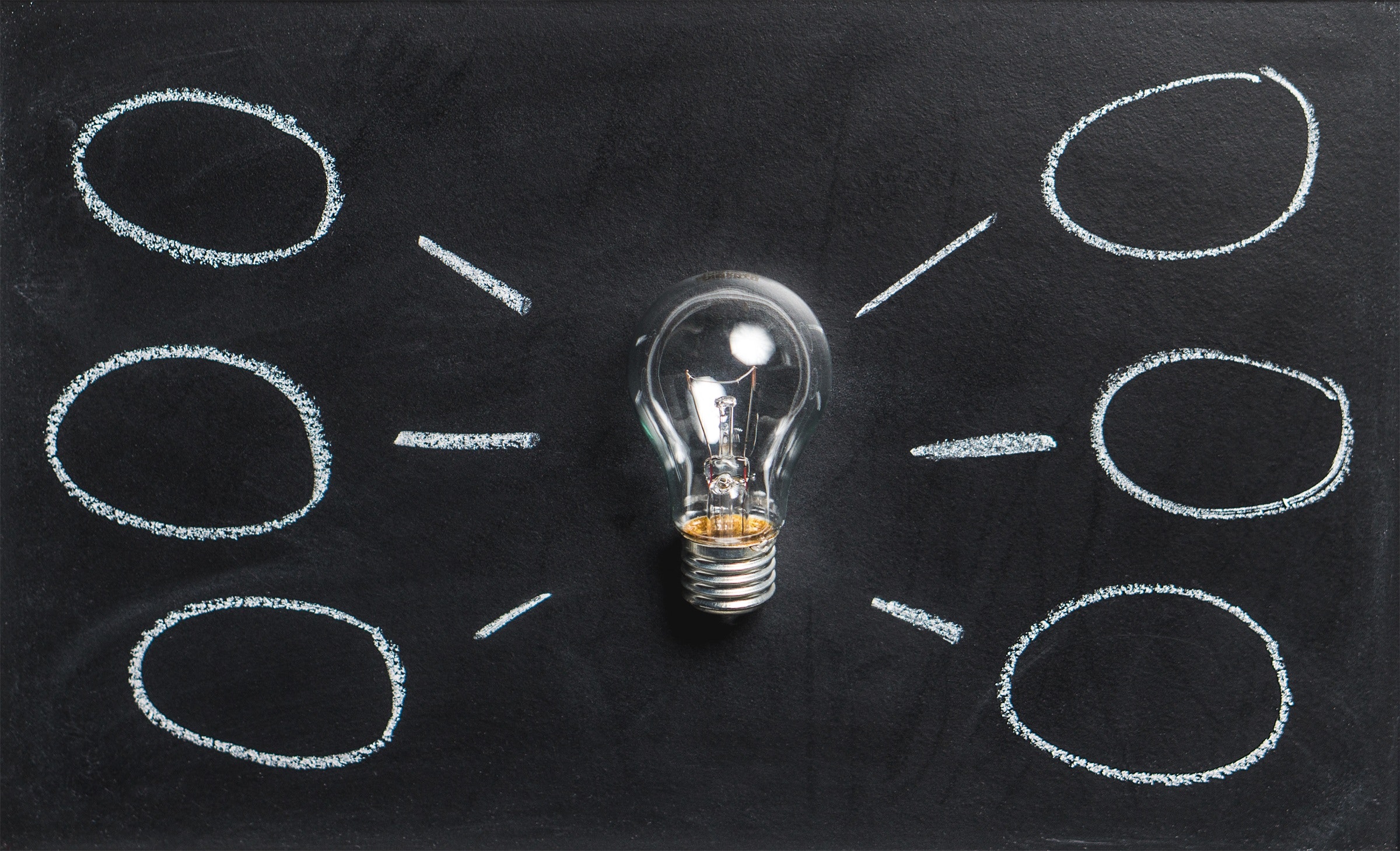
Detect areas of weakness to drive improvement
Compared to a single regression model, this cluster-and-regress approach improved the predictive performance by 5%. In addition, we generated error metrics to benchmark the performance in each cluster against that on the whole dataset to identify areas of weakness or strength.
As an illustrative example, the tree below shows that the predictions were particularly problematic for products with higher than average demand in the past quarter and longer than average lead time. This allows a tailored and direct process of improvement, such as collecting additional data or engineering new features that are better-suited for making predictions in this cluster.
This process of targeted intervention worked well for this Fortune 100 company and enabled rapid and efficient improvements in the model quality.
Unique Advantage
Why is the Interpretable AI solution unique?
-
End-to-End Interpretability
Full transparency in each step of the prediction pipeline increases data scientist efficiency
-
Better Communication
Enable business stakeholders to check prediction logic and verify intuition
-
Enhanced Performance
Training regressions in each cluster allows more flexible and stronger models
-
Useful Insights
Automatically highlight understandable areas that need improvement