Marketing Recommendations that Maximize Fund Flow
Personalized marketing strategies learned from historical interactions and outcomes in investment management
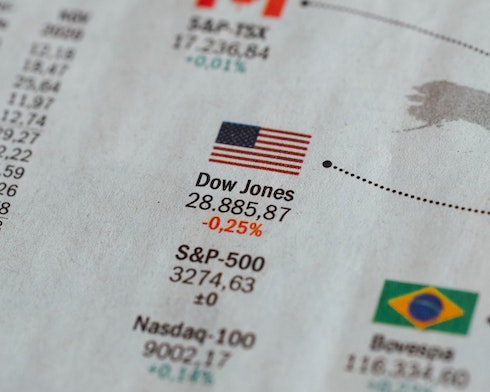
Marketing strategies in investment management are largely one-size-fits-all
Traditional approaches to marketing involve developing campaigns based on customer segments. This segmentation is often based on a clustering analysis using demographic information, or predictive models that estimate the success rates of marketing efforts based on customer information.
When considering how best to allocate marketing funds, these approaches fall short of our real goal, which is determining which marketing approach is best for each individual. This is a difficult task, as the historical data only tells us how an individual responded to the marketing approach that was used. Crucially, we do not know what would have happened had a different approach been used, as the data is only observational.
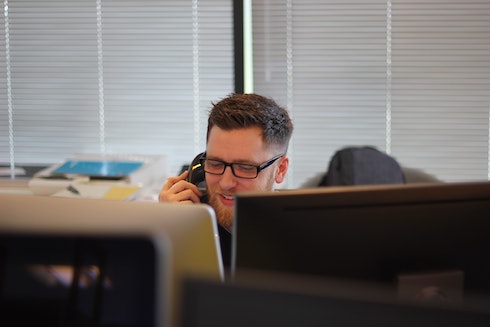
Marketing management tool based on what worked in the past
-
Categorize shared patterns under each marketing channel
Based on large volume of historical interactions data, the algorithm automatically segments customers into cohorts with similar responses to the various marketing channels, which are needed in the optimization step.
-
Meaningful cohort definitions
Each leaf of the tree creates an explainable segment of customers that have similar responses to prior marketing efforts. The interpretability of the tree allowed the marketing department to validate these segments against their intuition.
Illustrative Optimal Prescriptive Tree for Marketing in Investment Management
Increase in inflow via optimization
In a pilot study under the recommendation, the client estimated a lift of 8-15% in fund flows using the recommendations without increasing their marketing budget. In addition, the client identified a few cohorts where it can focus its effort on improving fund performance where marketing has limited impact.
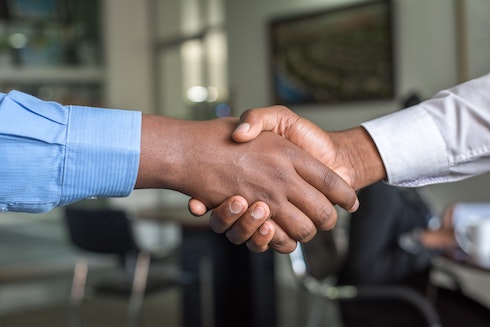
Unique Advantage
Why is the Interpretable AI solution unique?
-
Flexibility of Optimal Trees
Optimal Prescriptive Trees learns a highly accurate rule to predict and recommend at the same time
-
Actionable with validated insights
The model outputs direct action per customer, which was validated by marketing experts because of the model transparency
-
Working with the lack of counterfactuals
Even without knowing what happened under alternative marketing channels, the model can learn relevant effects with high accuracy
-
Integrate with formal optimization engine
Rather than predicting everything and optimizing, the model learns the necessary inputs for the optimization problem directly