Monitoring Hard Drives in a Data Center
Understanding how and why hard drives fail by monitoring their behavior
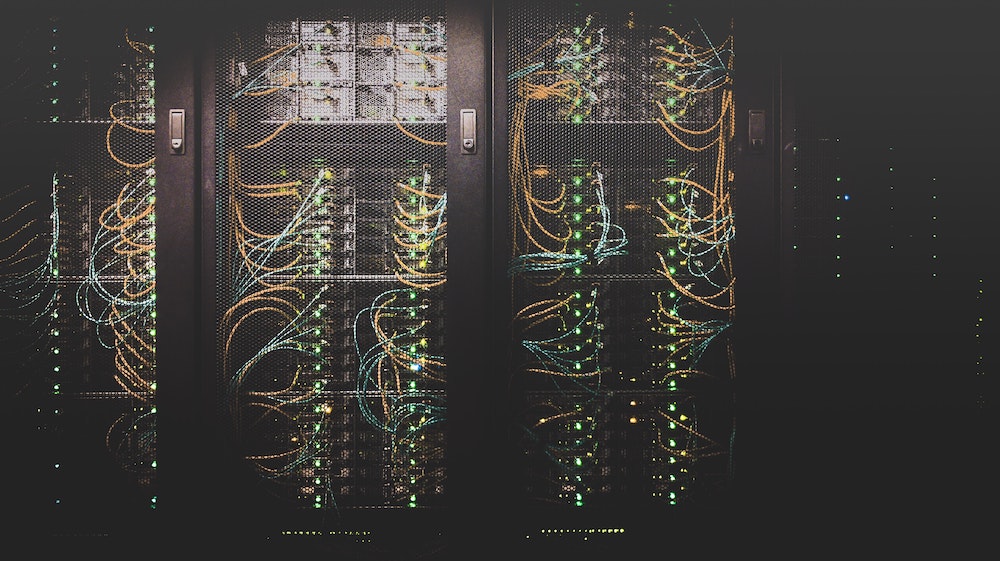
Correlation analyses are too simplistic
To optimize the data center's operation, workers need to know in advance which hard drives are likely to fail. Backblaze has identified five SMART metrics indicating impeding failure from univariate correlation analyses between individual SMART metrics and failure rates, as well as their workers' experience.
Despite being interesting, these findings are overly simplistic and not immediately actionable. What insights can we get using interpretable machine learning models?
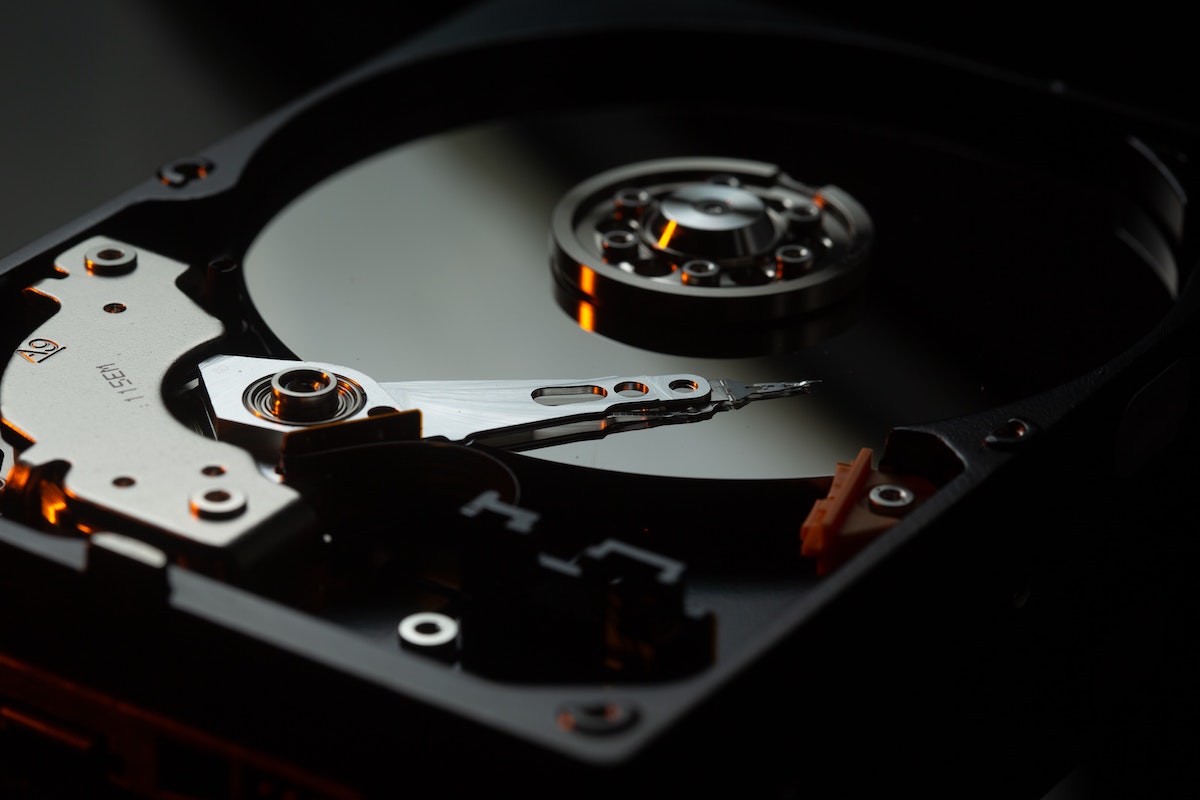
Understanding the overall health of a hard drive
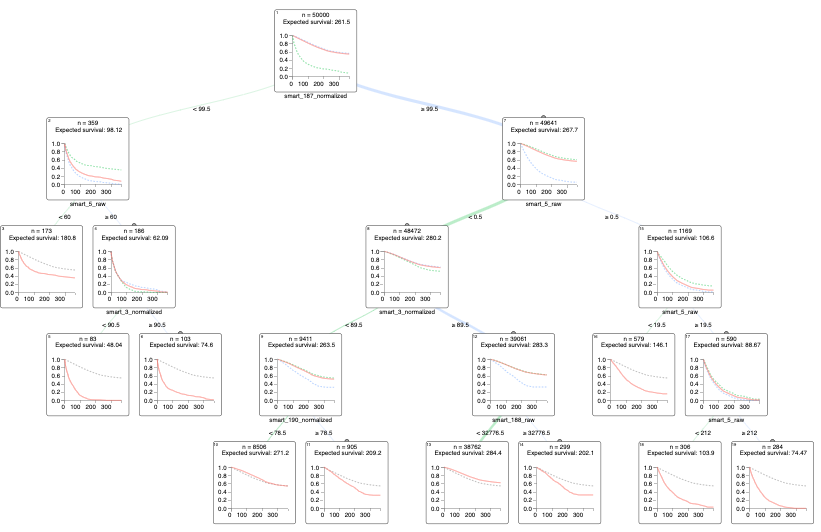
Predicting short-term failures
The end model can be easily visualized and understood by non-technical people, without sacrificing performance.
Our work was featured in a recent blog post by Backblaze and we presented the analysis at a webinar hosted by Backblaze.
Unique Advantage
Why is the Interpretable AI solution unique?
-
Detecting interpretable paths to Failure
Optimal Trees can automatically display paths to failure, as well as healthy behaviors, featuring correlations between several SMART metrics simultaneously
-
Specialized models for specific tasks
Depending on the question we are trying to answer, e.g. overall health monitoring, or predicting failure within a given fixed time window, we can choose between Optimal Survival Trees and Optimal Classification Trees
-
Adaptable to low data scenarios
If easily accessible data is scarce and comes from a short time frame, interesting findings can still be found using Interpretable AI’s software modules