Detecting Bias in Jury Selection
Investigating the presence of human biases in decision making with interpretable methods
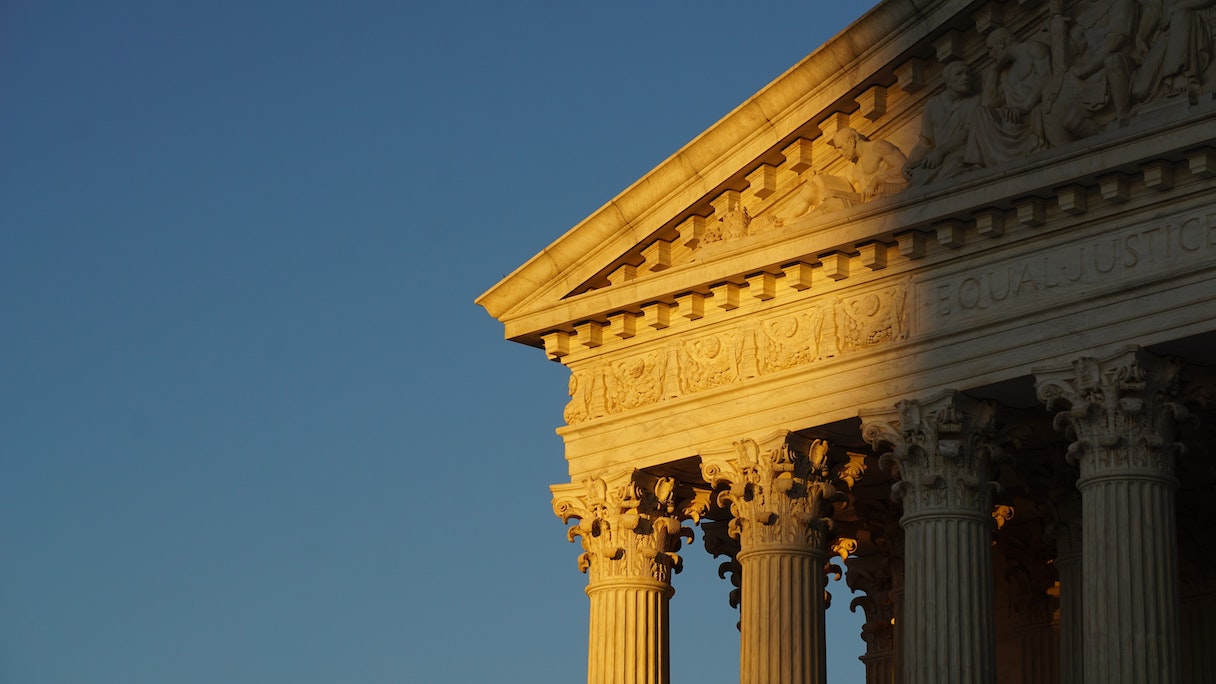
Was there bias in jury selection in Mississippi?
In 1986, the U.S. Supreme Court ruled that using race as a reason to remove potential jurors is unconsititutional. Despite this ruling, a large disparity in juror strike rates across races appears to remain. This disparity is the center of the 2019 U.S. Supreme Court case "Flowers v. Mississippi", where it was ruled that the District Attorney Doug Evans from the Fifth Circuit Court District in Mississippi had discriminated based on race during jury selection in the six trials of Curtis Flowers.
To support the case, APM Reports collected and published court records of jury strikes in the Fifth Circuit Court District and conducted analysis to assess if there was a systematic racial bias in jury selection in this district. As part of their analyses, they used a logistic regression model and concluded that there was significant racial disparity in jury strike rates by the State, even after accounting for other factors in the dataset.
To support the case, APM Reports collected and published court records of jury strikes in the Fifth Circuit Court District and conducted analysis to assess if there was a systematic racial bias in jury selection in this district. As part of their analyses, they used a logistic regression model and concluded that there was significant racial disparity in jury strike rates by the State, even after accounting for other factors in the dataset.
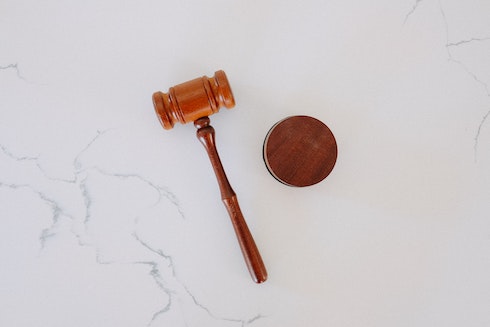
Race consistently identified as important with optimal models
Instead of the backward stepwise logistic regression model used in the original study, which iteratively selects and removes variables that are insignificant, we used Optimal Feature Selection, which automatically picks the optimal set of variables in a single step.
With Optimal Feature Selection, we still find that race is consistently selected as the most predictive variable of juror strike outcome at all levels of sparsity. This strengthens the conclusion in the original study, as we knowing that the variable selection is exact.
Furthermore, we observe a significant decrease in model performance when the race variable is removed, indicating this feature is providing unique signal in the data.
With Optimal Feature Selection, we still find that race is consistently selected as the most predictive variable of juror strike outcome at all levels of sparsity. This strengthens the conclusion in the original study, as we knowing that the variable selection is exact.
Furthermore, we observe a significant decrease in model performance when the race variable is removed, indicating this feature is providing unique signal in the data.
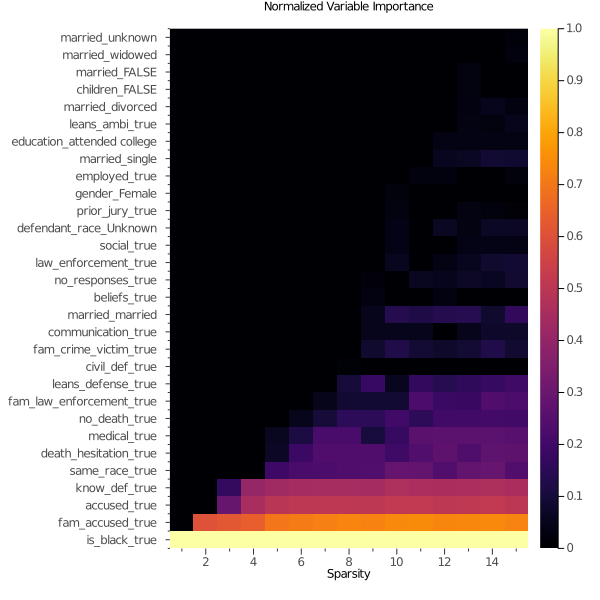
Variable importance across all sparsity levels from Optimal Feature Selection
Some subgroups have more prominent racial disparity
In addition to assessing overall bias, we aimed to identify subgroups with significant racial disparity. We trained an Optimal Classification Tree and conducted statistical tests between black and non-black jurors in each subgroup. We obtained two groups where there were no differences (those that have been accused of a crime, or have reservation about death penalty).
The remaining groups show significant disparity. For example, among jurors that have not been accused of crimes but know the defendant, there is an over 60% difference in struck rate between black and non-black.
These subgroups suggest systemic patterns of racial bias in the strike process, and provide direct characterizations of the situations in which black jurors are likely to have experienced discrimination.
The remaining groups show significant disparity. For example, among jurors that have not been accused of crimes but know the defendant, there is an over 60% difference in struck rate between black and non-black.
These subgroups suggest systemic patterns of racial bias in the strike process, and provide direct characterizations of the situations in which black jurors are likely to have experienced discrimination.
Optimal Classification Trees showing the racial disparity in different subgroups
Unique Advantage
Why is the Interpretable AI solution unique?
-
Optimal Selection
Exact variable selection strengthens the conclusion that race is highly improtant
-
Automatic Subgroup Identification
Optimal Trees automatically identifies subgroups where the racial disparity is the strongest